Unlocking Infinite Context Lengths for Large Language Models: The EM-LLM Breakthrough
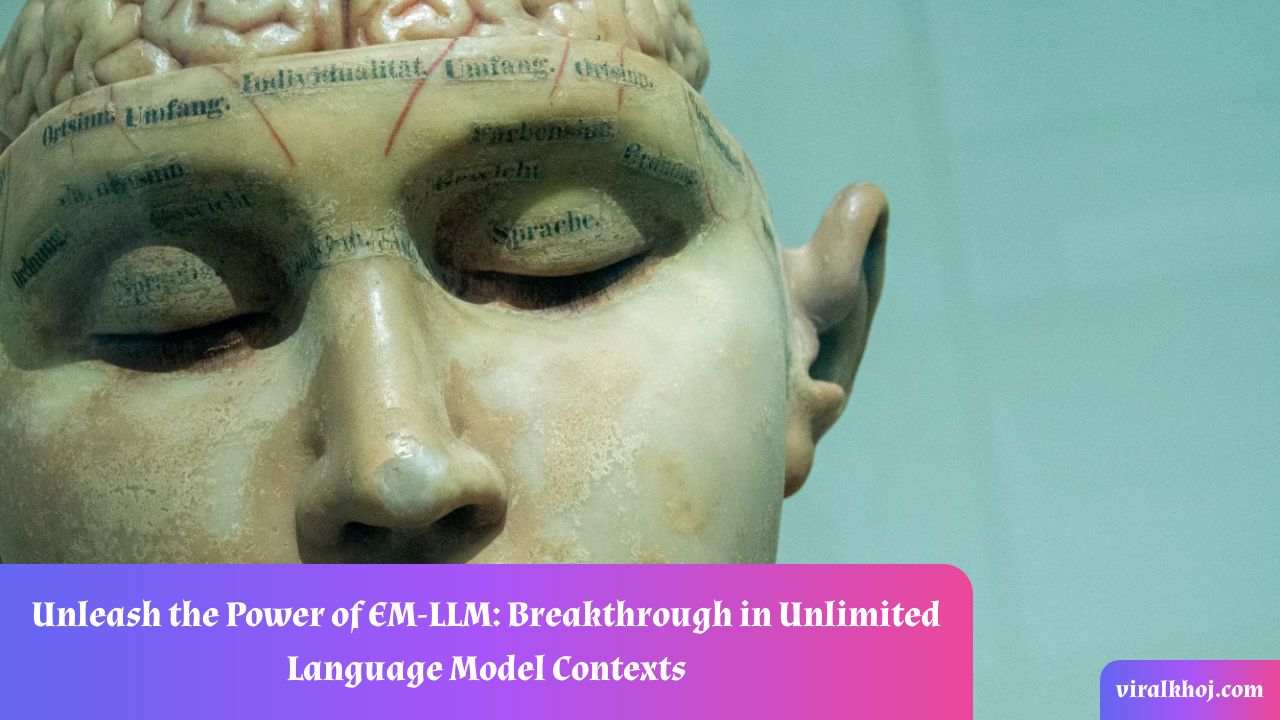
Unlocking Infinite Context Lengths for Large Language Models: The EM-LLM Breakthrough
Language models have revolutionized the field of natural language processing, enabling computers to understand and generate human-like text. However, one major limitation of large language models (LLMs) is their limited context length. This means that they can only consider a fixed number of preceding words or sentences when generating text, which can limit their understanding and coherence.
Addressing this challenge, a team of researchers from Huawei and University College London has made a significant breakthrough in emulating human episodic memory to enhance LLMs. In their paper titled "Human-like Episodic Memory for Infinite Context LLMs," they introduce EM-LLM (Episodic Memory-Large Language Model), a novel approach that integrates important aspects of episodic memory and event cognition into LLMs.
EM-LLM leverages insights from cognitive science to recreate human episodic memory within language models. By organizing tokens into episodic events using Bayesian surprise and graph-theoretic boundary refinement, EM-LLM enables LLMs to have potentially infinite context lengths without compromising their regular functioning.
Benefits of EM-LLM
One of the most intriguing aspects of EM-LLM is its scalability. Unlike other methods that rely on increasing computing power, EM-LLM provides a scalable computational framework that can handle long-context tasks without additional computational resources. This breakthrough not only enhances the performance of LLMs on long-context tasks, but it also opens up possibilities for testing hypotheses about human memory.
Furthermore, EM-LLM outperforms other proposals aimed at improving context windows for LLMs. When compared to InfLLM, EM-LLM demonstrated an overall improvement of 4.3%. In the Passage Retrieval task, EM-LLM achieved an impressive 33% improvement over InfLLM. This indicates that EM-LLM's event segmentation closely aligns with human-perceived events, bridging the gap between artificial systems and their biological counterparts.
Unlocking Infinite Context Lengths
The ability to unlock infinite context lengths in LLMs has significant implications for various applications. With longer context lengths, LLMs can better understand and generate text, resulting in more coherent and contextually relevant outputs. This can greatly enhance natural language understanding, machine translation, chatbots, and many other language-related tasks.
Moreover, the EM-LLM breakthrough paves the way for advancements in human-computer interaction. By emulating human episodic memory, LLMs can better mimic human-like information retrieval and access. This could lead to more intuitive and efficient interactions with language models, making them even more valuable tools for research, education, and everyday use.
Continued Research and Industry Impact
Unlocking infinite context lengths is a topic of intense research interest, with major companies like Google and Meta also exploring this area. The ongoing efforts to increase context lengths demonstrate the importance of pushing the boundaries of language models and their applications.
As the field continues to evolve, EM-LLM stands out as a groundbreaking approach that combines cognitive science and machine learning. Its success in emulating human episodic memory and achieving superior performance in long-context tasks marks a significant step forward in the development of advanced language models.
Overall, the EM-LLM breakthrough provides a promising glimpse into the future of language models and their potential to understand and generate text with infinite context lengths. By bridging the gap between artificial systems and human memory, EM-LLM opens up new possibilities for enhancing language understanding and human-computer interaction.
Discover more about the EM-LLM breakthrough and its implications for language models in the full paper by Huawei and University College London.
For more insights and updates on the latest advancements in artificial intelligence and machine learning, visit MachineCon.
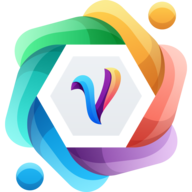
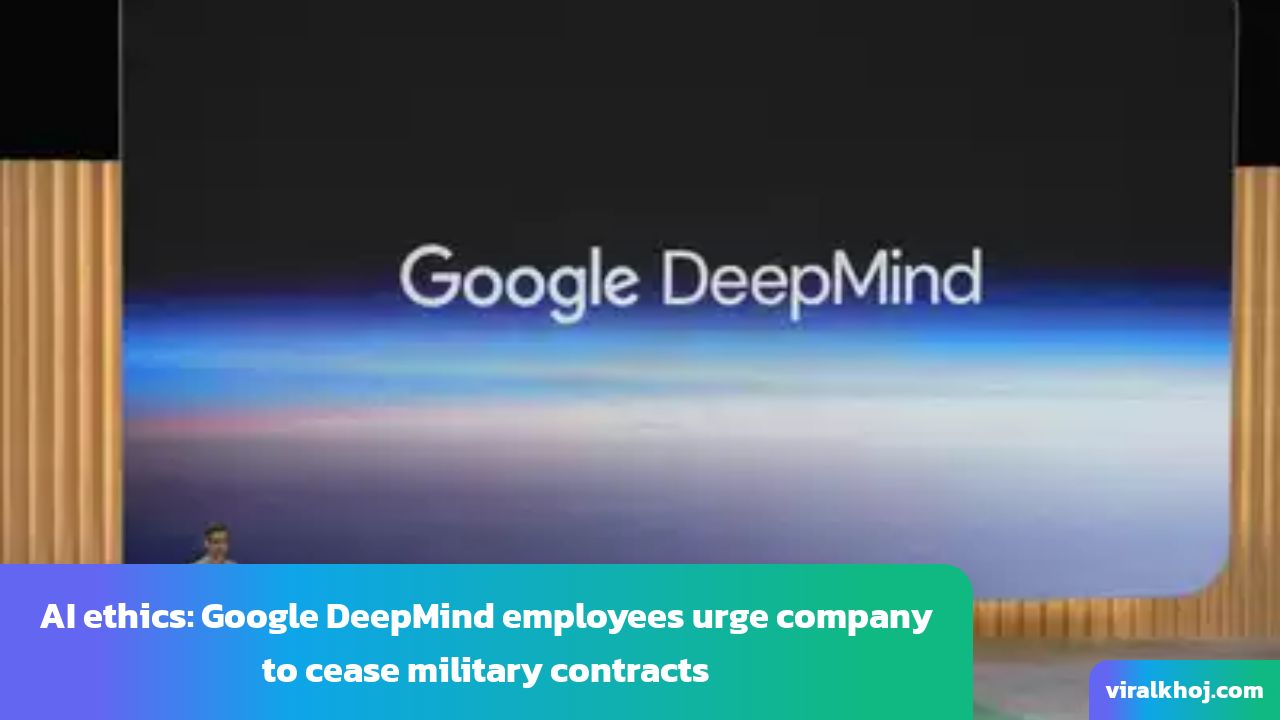
The Ethical Implications of AI in Warfare: A Call for Responsibility
27 Aug 2024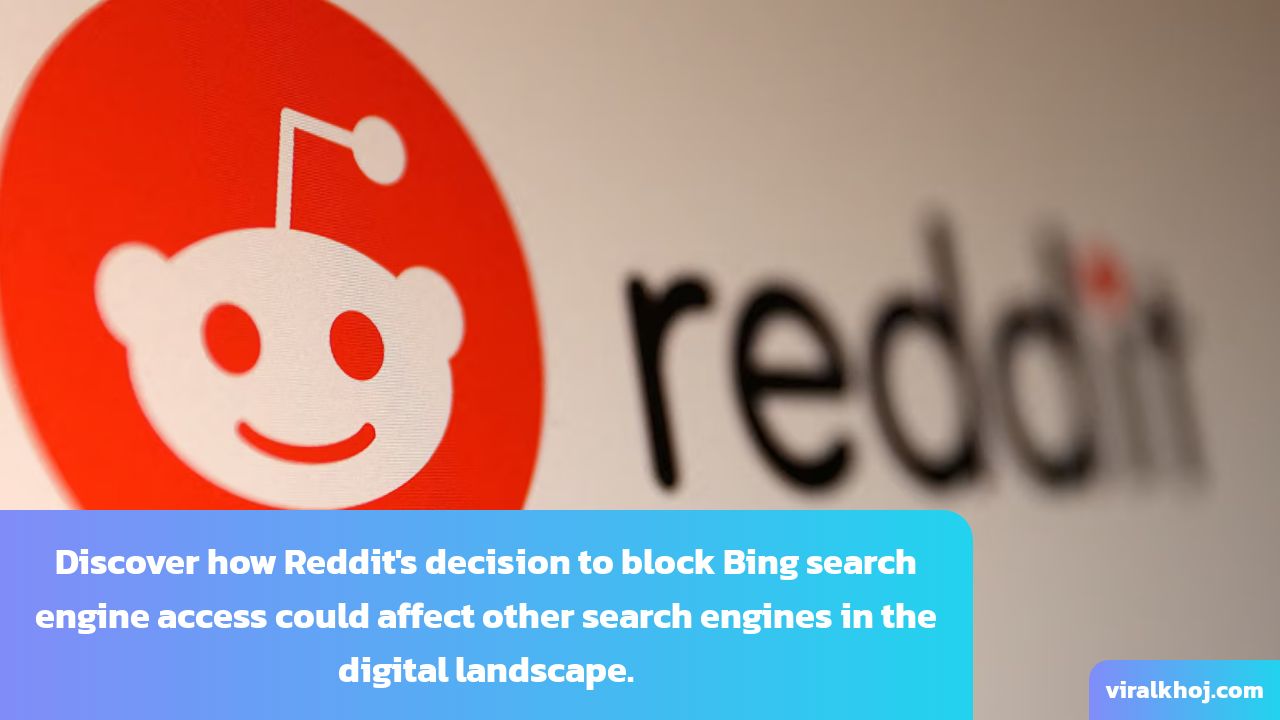
Reddit Blocks Bing Search Engine Access: Impact on Other Search Engines
28 Jul 2024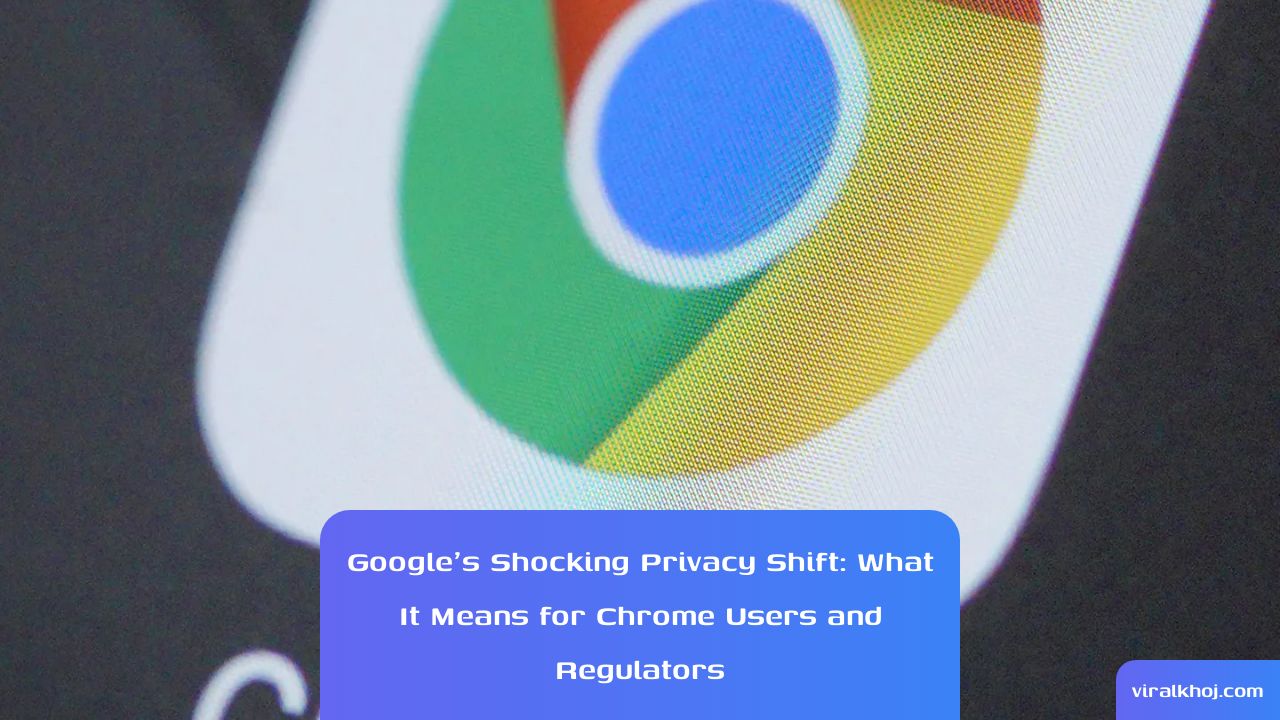
Google's Privacy Shift: Implications for Chrome Users and Regulators
28 Jul 2024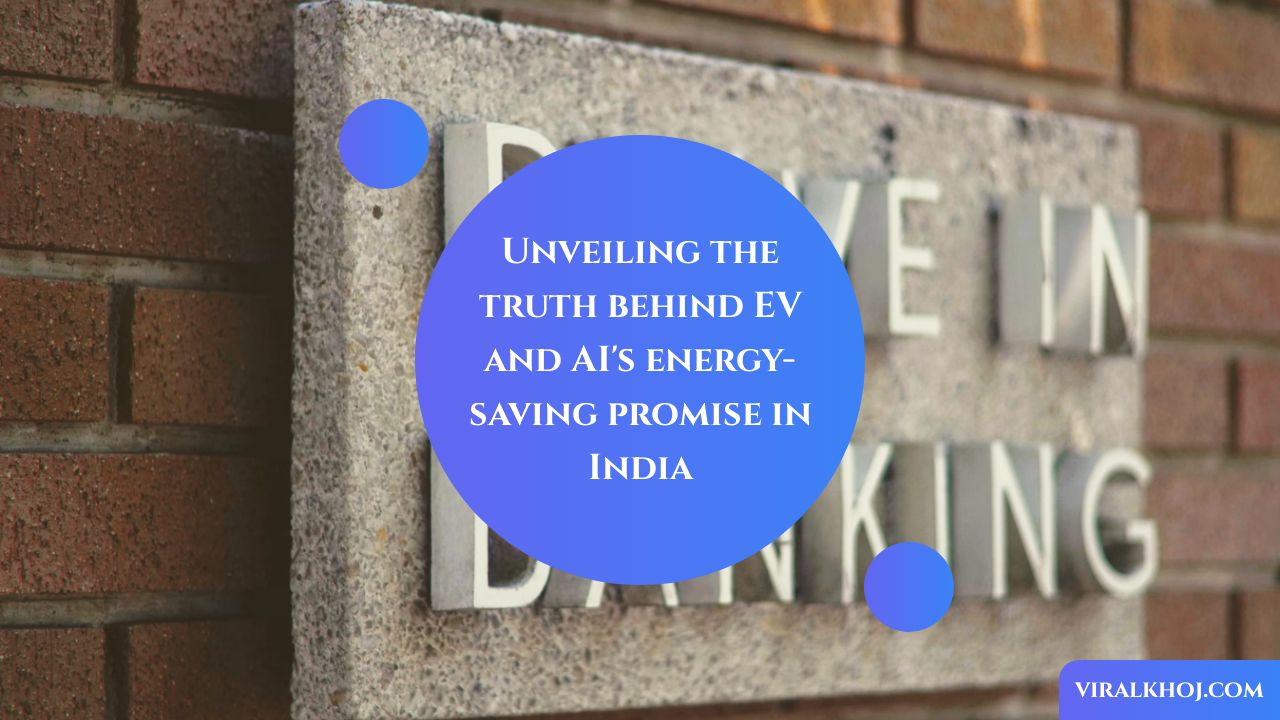
Why EV and AI’s energy-saving pitch in India is a clickbait
17 Jul 2024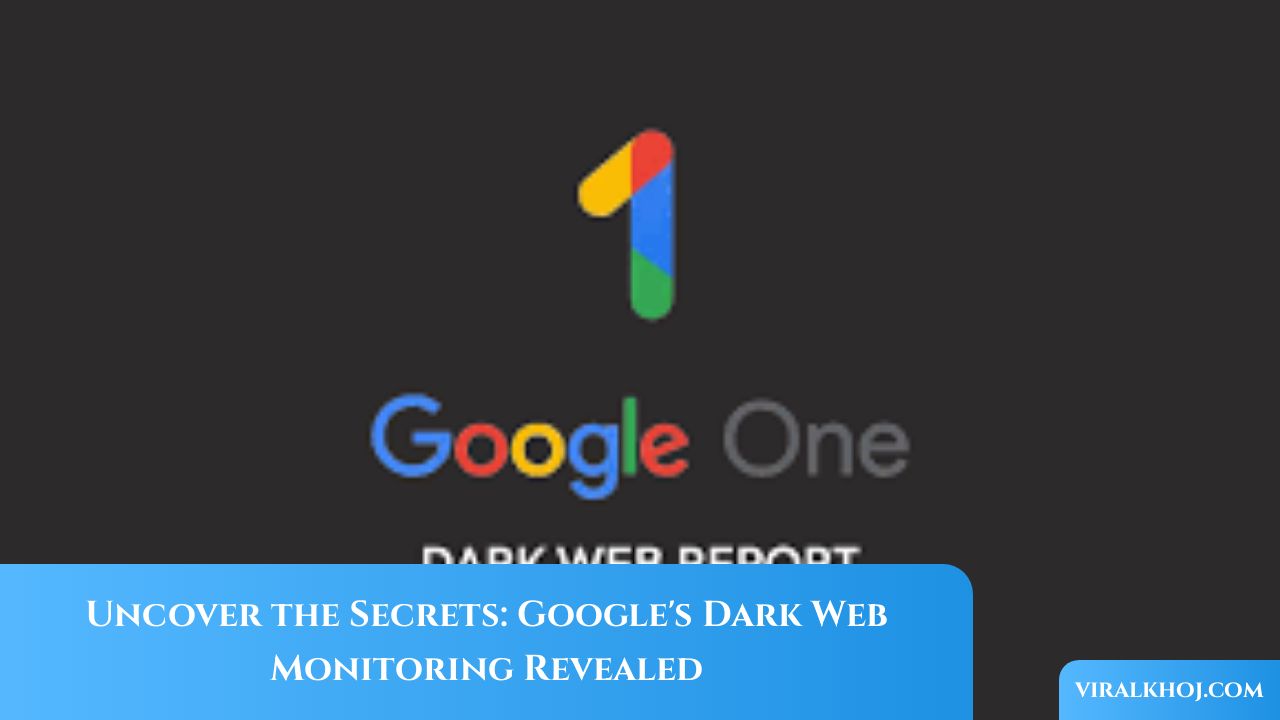
Google Dark Web Monitoring: What You Need to Know
11 Jul 2024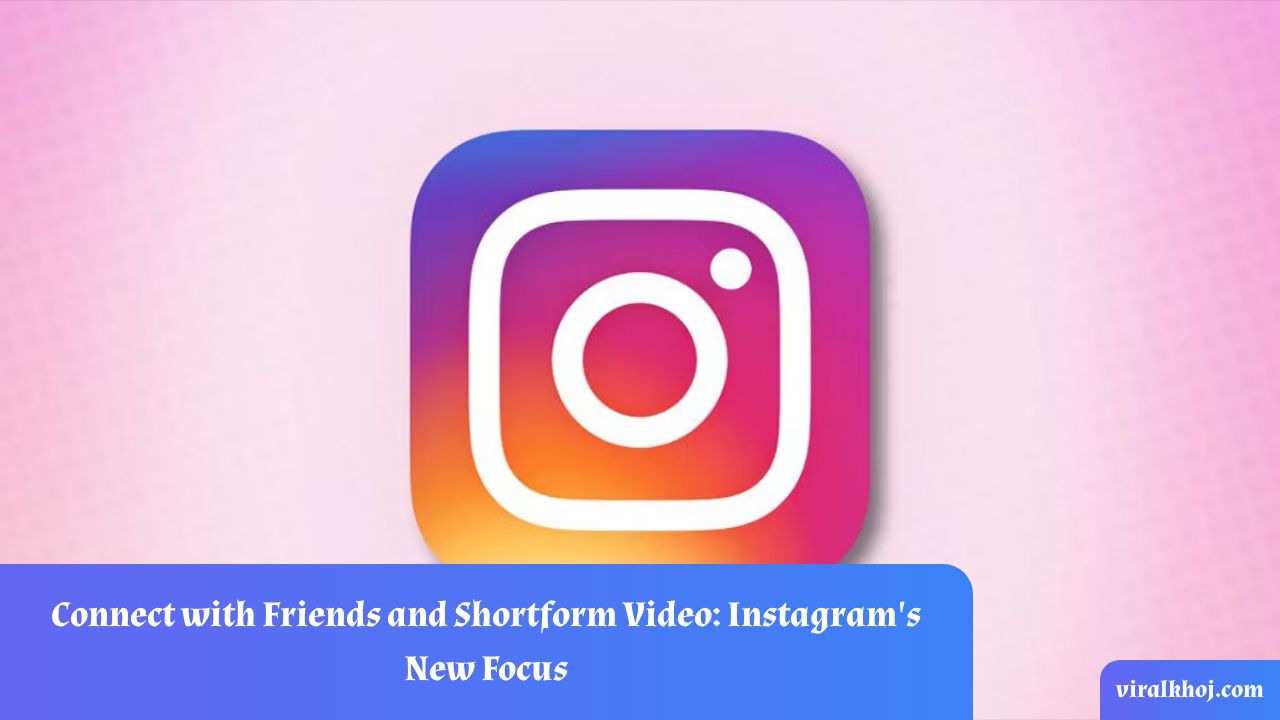